Understanding Image Annotation for Machine Learning
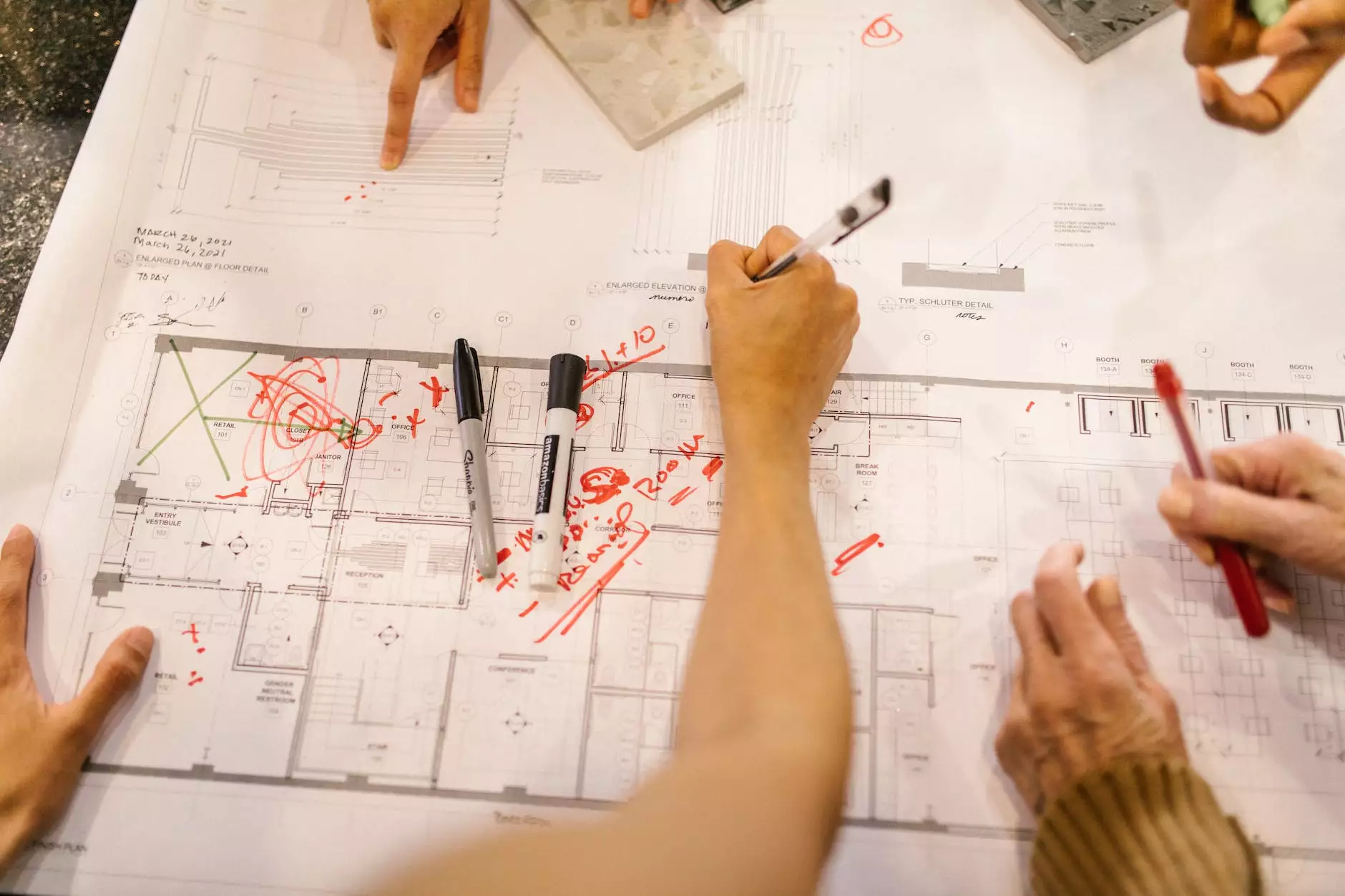
Image annotation for machine learning is a pivotal process that enhances the learning capabilities of artificial intelligence (AI) models. In the evolving landscape of machine learning and deep learning, understanding this concept is essential for those seeking to develop sophisticated AI applications. This article will explore the nuances of image annotation, its importance, and how you can leverage the best tools and platforms to optimize your machine learning projects.
The Importance of Image Annotation in Machine Learning
Machine learning relies heavily on data, and image annotation is a critical step in preparing images for algorithmic learning. By labeling images accurately, organizations can train models that recognize and predict outcomes effectively. This process is not merely a technical step; it forms the foundation of a successful AI application. Here’s why image annotation is vital:
- Data Quality: Quality annotated data leads to more accurate and reliable machine learning models.
- Model Performance: Well-annotated images improve the performance of your AI by reducing the likelihood of errors during prediction.
- Scalability: As you scale your AI applications, consistent image annotation ensures that your models can handle diverse data inputs.
- Industry Applications: Different industries, from healthcare to automotive, rely on precise image annotations for tasks such as medical imaging analysis, object detection, and autonomous driving.
Types of Image Annotation Techniques
When it comes to image annotation for machine learning, several techniques can be employed, depending on the data and the specific goals. Here are the most prevalent methods:
1. Bounding Box Annotation
This is one of the most basic forms of image annotation. It involves drawing rectangles around objects in an image. This method is commonly used in object detection tasks.
2. Semantic Segmentation
Semantic segmentation classifies each pixel in an image into a specific category. This technique is essential for tasks that require a detailed understanding of the spatial structure in images.
3. Polygonal Annotation
This technique allows for more precise annotations compared to bounding boxes, especially for objects with irregular shapes. A polygon is drawn around the object to provide an exact outline.
4. Landmark Annotation
Landmark annotation involves marking specific points on an object. This method is widely used in facial recognition and gesture analysis.
5. Full Image Annotation
This involves labeling the entire image with a single tag. It is often used in image classification tasks where the goal is to categorize an image as a whole.
Choosing the Right Data Annotation Tool
With various annotation techniques available, selecting the right data annotation tool is crucial for the success of your machine learning project. Here are essential features to consider:
- User-Friendly Interface: The tool should be intuitive and easy to navigate, allowing annotators to work efficiently.
- Support for Various Annotation Types: Choose a tool that accommodates different annotation methods suitable for your project.
- Collaboration Features: Look for platforms that allow multiple users to collaborate seamlessly on annotation tasks.
- Integration Capabilities: The ability to integrate with machine learning frameworks and models can streamline your workflow.
- Quality Assurance Mechanisms: A good tool should have built-in quality control features to maintain the accuracy of annotations.
Keylabs.ai: A Leader in Image Annotation Solutions
At Keylabs.ai, we specialize in providing top-notch image annotation services tailored to your machine learning needs. Our platform offers advanced data annotation tools designed to deliver high-quality annotations efficiently. Here’s why Keylabs.ai stands out in the competitive landscape:
1. Advanced Annotation Tools
Our data annotation platform is equipped with cutting-edge technology that allows for precise and scalable image annotations. Whether you require bounding boxes, semantic segmentation, or polygonal annotations, we have you covered.
2. High-Quality Assurance
We prioritize quality at Keylabs.ai. Our rigorous quality assurance processes ensure that every annotation meets industry standards, providing you with reliable data for your machine learning models.
3. Collaborative and Flexible Services
Our platform enables teams to collaborate effectively, making it easier to manage large-scale annotation projects. You can track progress and ensure that deadlines are met with our flexible project management features.
4. Custom Solutions
Understanding that each project is unique, we offer customized annotation solutions tailored to fit your specific needs and objectives. Our team works closely with you to design a workflow that aligns with your goals.
Steps to Implement Image Annotation in Your ML Workflow
Integrating image annotation into your machine learning workflow can be broken down into several steps:
Step 1: Define Your Goals
Start by clearly defining the objectives of your machine learning project. Identify what you want the model to learn and the type of annotations required.
Step 2: Choose the Right Annotation Method
Based on your goals, select the most suitable image annotation technique. This will guide your concrete project requirements.
Step 3: Select an Annotation Tool
Choose a robust data annotation tool, like those offered by Keylabs.ai, that aligns with your project needs.
Step 4: Prepare Your Dataset
Gather and preprocess your images. Ensure image quality is optimized to facilitate accurate annotations.
Step 5: Start Annotating
Begin the annotation process using your chosen tool. Ensure that the annotation team is well-versed in the required techniques to maintain consistency.
Step 6: Quality Control
Implement quality control measures to review annotations for accuracy. This could involve peer reviews or automated checks using machine learning algorithms.
Step 7: Training Your ML Model
Once the annotations are complete, you can use the labeled data to train your machine learning model. Monitor performance and make adjustments as necessary.
Future Trends in Image Annotation and Machine Learning
As technology continues to evolve, so does the field of image annotation. Here are some future trends that may shape the industry:
- Automated Annotation: Advances in AI are making it possible for machines to perform annotation tasks with minimal human intervention, potentially speeding up the process and reducing costs.
- Improved Quality Assurance: New methodologies and tools will likely emerge to enhance quality control in image annotation, ensuring even higher accuracy rates.
- Enhanced User Experience: Future annotation tools will prioritize user experience, integrating intuitive interfaces and AI-driven features for ease of use.
- Integration of Augmented Reality (AR): The incorporation of AR in annotation processes can provide more interactive and effective annotation experiences.
Conclusion
In conclusion, image annotation for machine learning is an indispensable part of developing high-performing AI models. By choosing the right techniques and tools, such as those offered by Keylabs.ai, organizations can significantly enhance their machine learning outcomes. As you embark on your machine learning journey, investing in quality image annotations will set a strong foundation for success.
For tailored data annotation solutions and to learn more about our data annotation tools and platforms, visit Keylabs.ai today!